5 Myths about Data
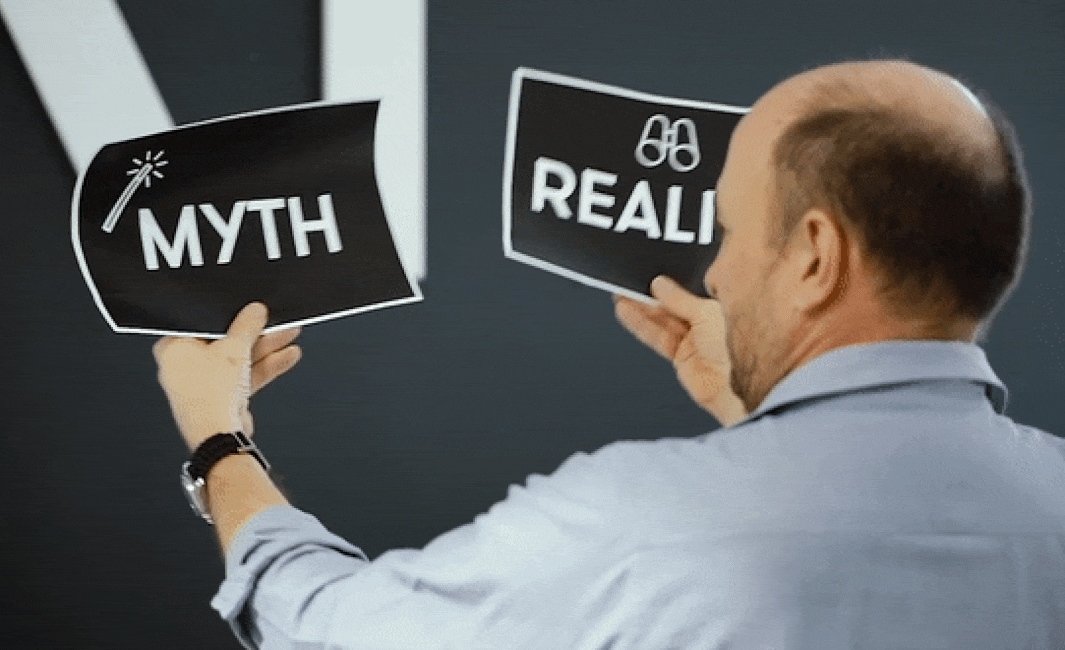
It is clear that data has become the most important asset of almost every company. Those that have spent decades in the trenches of database design, architecture and usage have seen the growth of this awareness explode in the last decade. But along with increased visibility, new technologies have driven a need to revise many of the traditional narratives that held true in previous times. In this brief, some of these myths will be explored and hopefully challenge to make room for new approaches and techniques to deliver data-driven insight and profitability for the enterprise.
1. All data must be stored.
Not all data has value. The most important data is the data that drives actionable insight. Many projects have assumed this means you must collect everything and then derive the insight from complex algorithms. While there may be information gleaned from broad data collection, it is better to start with a defined problem to solve and then work back to the data required to illuminate it. This process alone will teach your data management organization about volume, variety, velocity and cleanliness of the source data to be used in your projects. For example, there may be types of event data that only have value for the lifetime of the event, and their value diminishes rapidly over time. It would be a waste of resources to persist this data beyond that point. There may be other data that has value only after certain other events have transpired. This data must be carefully curated to preserve its integrity and accessibility until it is consumed. After these parameters are understood, there will be a much more reliable understanding of the most important data to be incorporated into the data architecture.
2. There is unstructured data.
All useful data has structure. While it may be un-modeled, there is always a structure to data that has value. For example, a seemingly unstructured text document has a structure. There are sentences, paragraphs, linguistic pattern, formatting and other attributes of text that can be modeled to include in a data architecture. Even complex streaming protocols like the ones used in manufacturing or vehicles can be modeled and consumed into a well-structured analytic consumption framework.
3. Data can predict the future.
Data alone does not have predictive value. In order to create behavioral models of future events, there must be a lot of data collected which applies to the type of problem that is being investigated. Even with voluminous amounts of data, without the proper model training and testing, there is little to be learned from the output of your calculations as they apply to the future. If this is the goal of the project, it is well-advised to engage with well-trained data scientists in the creation, population and analysis of the models to be tested.
4. A Data Lake is the Solution.
The data lake (a place for storing every possible data generated in your company) has become a popular feature of many architectures. While there may be a value to implementing a large multi-structured persistent storage mechanism in the overall data architecture, this is no guarantee that there will be consumers capable of using it to derive any meaningful insight. These data lakes have often just become network-based dumping grounds for data that is never again brought to the surface to meet the strategic goal of actionable insight. A more prudent approach is to create a hierarchy of data metrics, such as temperature or velocity of storage, and to develop the appropriate persistent target to meet the requirements derived from the appropriate classification. This may indicate the need for a location to store unstructured datasets, but that should not be the default position for all data within the system.
5. Big data is expensive.
With cloud-based platforms like Google, AWS, Azure and IBM Cloud, it is now possible to prototype and test data models and projects with little to no upfront investment. All of these providers deliver highly elastic services that can scale based not just on storage, but also on processing and velocity of data. This means that a system can be vetted for technical completeness and reliability at a very low cost.
How can MASSIVE ART help?
Developing and maintaining a Data Architecture (big or small) can be a daunting project. The data architecture and integration experts at MASSIVE ART have the experience and capacity to assess your current ecosystem and provide guidance towards the insight that can be revealed through a well designed data platform. If you have any questions, get in touch with our consultants to help you grow with these challenges and opportunities.
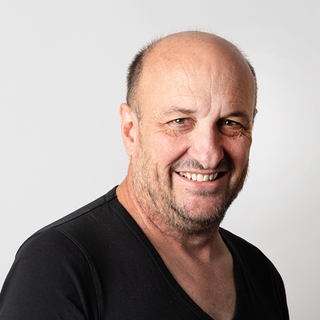